Databricks
Databricks is a unified data analytics platform built on Apache Spark. It provides a collaborative workspace for data scientists and engineers to work together on data projects. It supports data engineering, data science, machine learning, real-time analytics, and integrates with various tools and services. Databricks offers security and governance features to protect data and ensure compliance.
Azure Event Hub
Azure Event Hubs is a cloud-based event streaming platform provided by Microsoft Azure. It is a highly scalable and distributed event ingestion service that can handle and process large volumes of streaming data in real-time. Event Hubs is designed to simplify the collection, storage, and analysis of event data from a wide range of sources.
Prerequisite:
- Knowledge about Spark
- Knowledge about Streaming Data
- Resource to be created:
- Databricks Workspace
- Event Hub Namespace
- Event Hub
Architecture
Steps to Build a Streaming Pipeline on Databricks to ingest Data from event hub:
1. A Python program needs to be written to create or imitate streaming data flow.
2. Writing a Python program is necessary to produce the data required for toll collection.
3. Within our code, we’ll utilize Python libraries such as asyncio, random, datetime, json, and azure-eventhub.
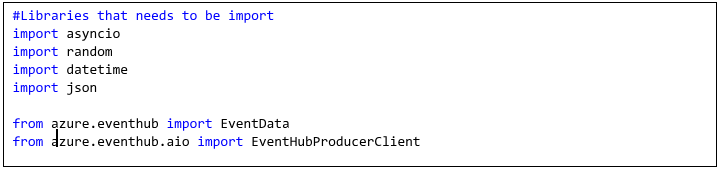
4. Within your code, make sure to include the authentication details for the event hub.

5. It’s important to create a function that generates the data and encapsulate it within an iterator to produce a substantial amount of data.
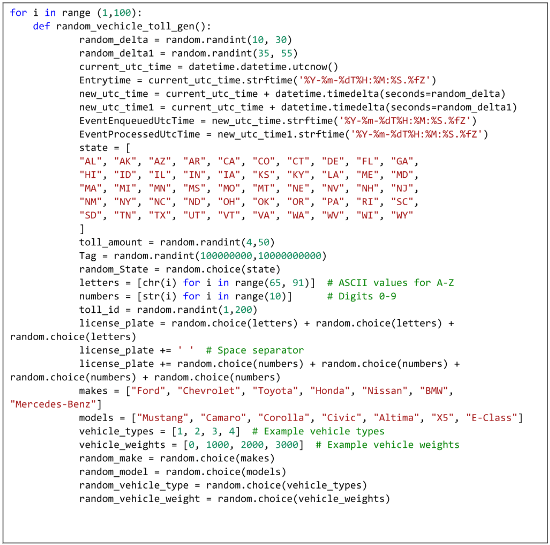
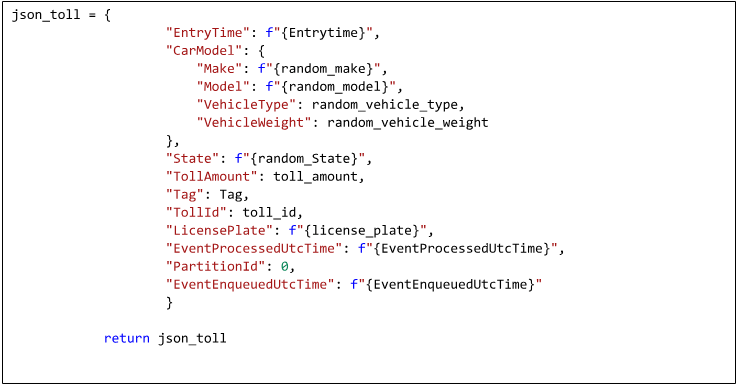
6. Next, we need to create an asynchronous function to send the data to the event hub. Additional configurations like consumer groups can be included in the code. For this purpose, the default consumer group has been utilized.
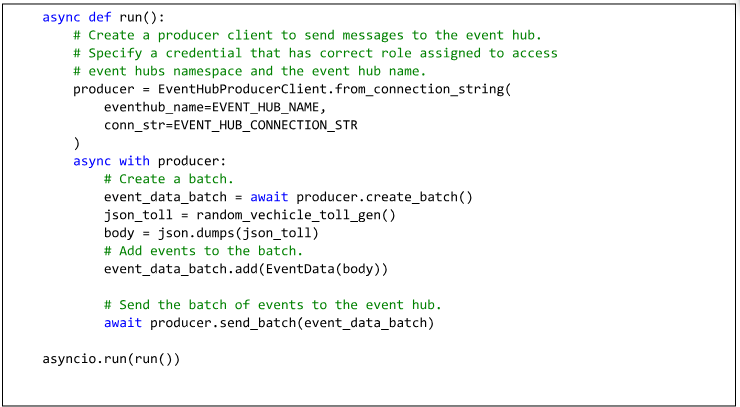
7. Following that, it’s essential to configure the Databricks cluster to install the Maven library necessary for executing our code.
Coordinates: com.microsoft.azure:azure-eventhubs-spark_2.12:2.3.15
Note spark_2.12 will depend on your Scala version if its 2.11 then replace 2.12 with 2.11
8. Our objective is to develop PySpark code that fetches data from the event hub, processes it, and writes it back to Delta Lake.
9. Initially, we must import the necessary libraries to achieve this goal.
10. Following that, we should proceed to define the schema for the streaming dataset from the source.
11. We will utilize the Read Stream method to ingest data from the Event Hub. Additional parameters can be provided depending upon requirements, please refer readStream documentation
12. In this case, the chosen format is “eventhubs,” and we have provided the necessary credentials via options. Furthermore, we’ve specified the specific content we are interested in.
13. The output represents the data acquired from the event hub. We have converted binary data into dataframe while reading the stream.
14. Subsequently, we proceed to flatten the data, extracting the desired columns and casting them into the appropriate data types. Following this, we select the specific columns that are needed for further processing.
15. Finally, we use the writeStream method to store the processed data into a Delta table. If needed, additional parameters can be specified based on the specific requirements. Please refer to writeStream documentation
16. By executing a query on the Delta Table, we can observe that the data has been successfully populated.